Comparative Analysis of Crash Simulation Results using Generative Nonlinear Dimensionality Reduction
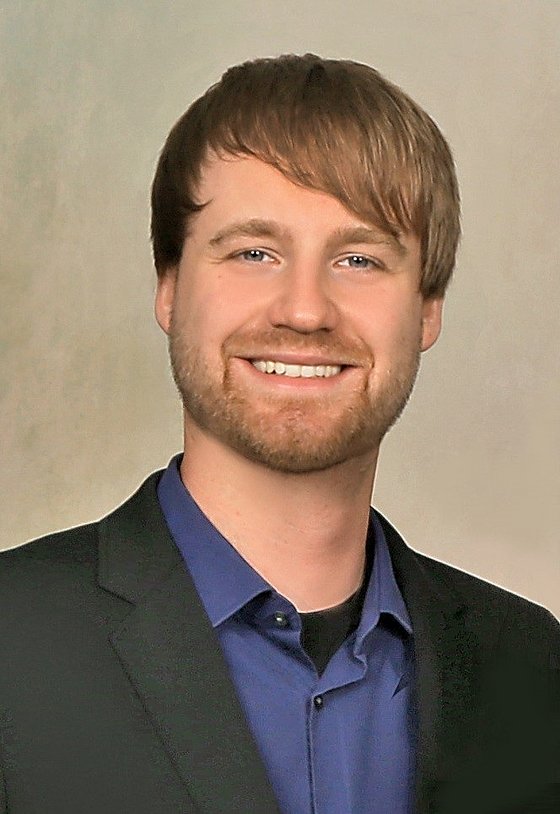
Numerical simulations are an integral part of today’s product development process. Analysing and comparing multiple simulation results is a time consuming but necessary step in utilising several results. Thus, it is important to develop methods which speed up this Comparative Analysis by identifying differences and commonalities in the results and provide means to visualise possible variances. Furthermore, it is crucial to accurately determine how these variances or similarities are related to each other. The so-called Dimensionality Reduction Methods (DRMs) have been used in extracting the underlying structure of variance in simulation results since several years. In recent years, the need for nonlinear reduction approaches has been shown in several applications. One widely used analysis method called Difference Principal Component Analysis (DPCA), which is specifically designed to compute the correlation between variation in different parts of the simulation results, is, however, based on a linear reduction approach. The aim of this dissertation is to extend the DPCA with nonlinear Dimensionality Reduction (DR), which has never been done until now. To achieve this aim, the two underlying steps of the DPCA’s analysis workflow were modified. For the first step of DR, several established methods of three classes of generative DRMs have been extended amongst others by importance factors to be used in this analysis. For the second so-called subtraction step, the new generalised concept of Difference Dimensionality Reduction was introduced. Two specific implementations of this general concept were implemented in this work, which can be combined with the different reduction methods in the first step. The newly developed methods were thoroughly tested on multiple examples in this work: Firstly, on artificial examples to test the individual steps in an isolated environment and secondly on simulation results to evaluate the method’s performance on realistic data sets. These new approaches were able to accurately determine the correlation between artificial data sets and provided better results for different parts of a set of simulation results compared to the state of the art. In the case of a nonlinear relation between these parts, the superiority over linear approaches was demonstrated in the evaluation, while underlying linear dependencies were also confirmed by the nonlinear methods. With the successful modifications done in this work, the DPCA’s workflow is now meaningfully applicable to data sets with nonlinear dependencies. While the linear variant was used in the context of crash simulations for several years, this application was questionable since it contains many nonlinearities. The newly developed variants remove this uncertainty, and the evaluation examples suggest a broad range of possible applications for these new methods, as nonlinearities can occur in many data sets, resulting for example from topology optimisation or parameter variation.